使用Vanna.ai通过GPT 4o与您的SQL数据库进行交流
Sure, here's the HTML structure with the text translated to simplified Chinese:
```html
创建一个使用OpenAI GPT 4o的文本到SQL管道
创建一个使用OpenAI GPT 4o的文本到SQL管道
```
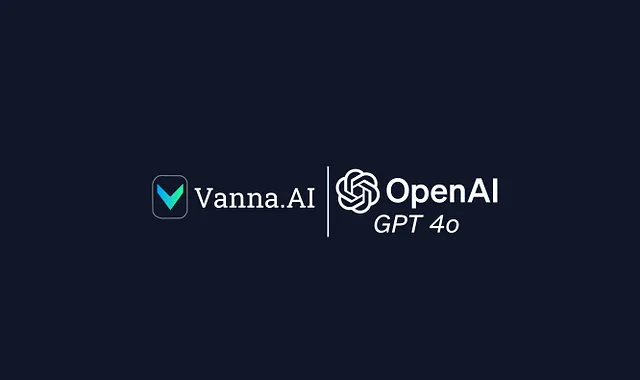
Sure, here is the translated text in simplified Chinese:
```html
在寻找能够解决与大型语言模型和数据科学相关问题的人?点击这里:
I can't directly access or translate content from external links. However, you can copy the English text from the form and provide it here, and I can help translate it into simplified Chinese for you.
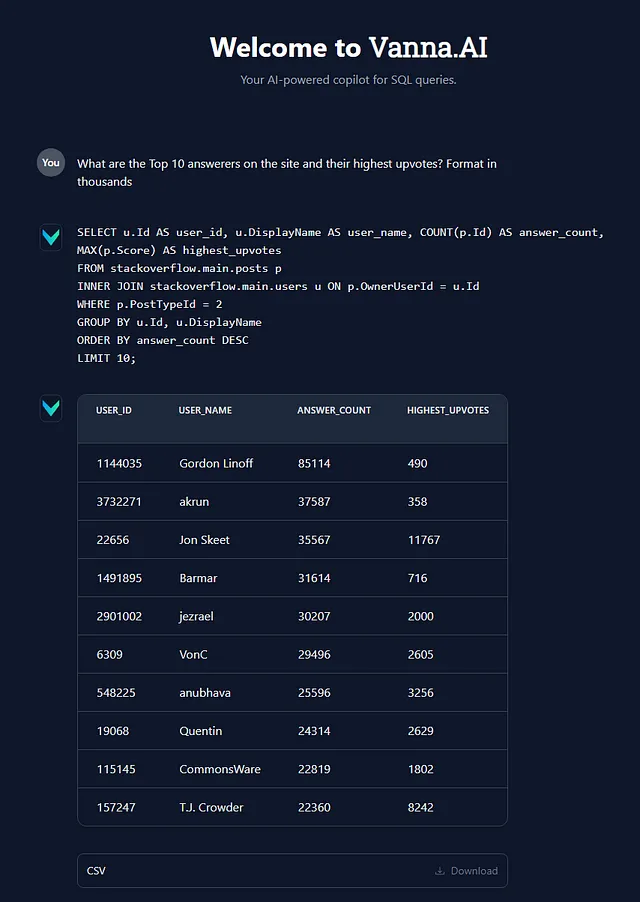
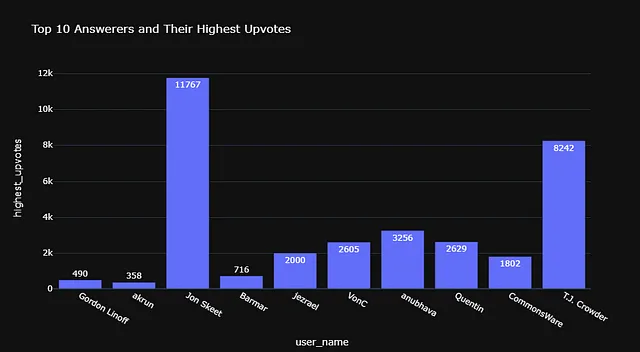
开始使用
from vanna.openai import OpenAI_Chat
from vanna.vannadb import VannaDB_VectorStore
class MyVanna(VannaDB_VectorStore, OpenAI_Chat):
def __init__(self, config=None):
MY_VANNA_MODEL = # Your model name from https://vanna.ai/account/profile
VannaDB_VectorStore.__init__(self, vanna_model=MY_VANNA_MODEL, vanna_api_key=MY_VANNA_API_KEY, config=config)
OpenAI_Chat.__init__(self, config=config)
# Add your OpenAI api_key
vn = MyVanna(config={'api_key': 'sk-...', 'model': 'gpt-4o'})
连接到数据库
Sure, here is the text translated into simplified Chinese while keeping the HTML structure: ```html
Vanna内置了这8个数据库的连接器(您可以通过添加几行额外的代码连接到其他数据库):
```- Sure, here is the translation:
```html
Postgres SQL```
- Sure, here's the translation:
```html
Oracle```
- Sure, here's the translation in simplified Chinese: ```html 鸭DB ```
- ```html
MySQL
``` - SQLite
- Certainly! The simplified Chinese translation of "Big Query" while keeping the HTML structure intact is: ```html 大查询 ```
- Sure, here's the translated text in simplified Chinese while keeping the HTML structure: ```html ```
- Microsoft SQL 微软 SQL
通过查阅文档,您可以了解如何连接您的特定数据库。就本文而言,我将连接到 DuckDB StackOverFlow 数据库。数据库可以在这里找到!
#This is how you can connect to a DuckDB database
vn.connect_to_duckdb(url='motherduck:[<database_name>]?motherduck_token=<token>&saas_mode=true')
Sure, here's the translation of "Training" into simplified Chinese while keeping the HTML structure: ```html
培训
```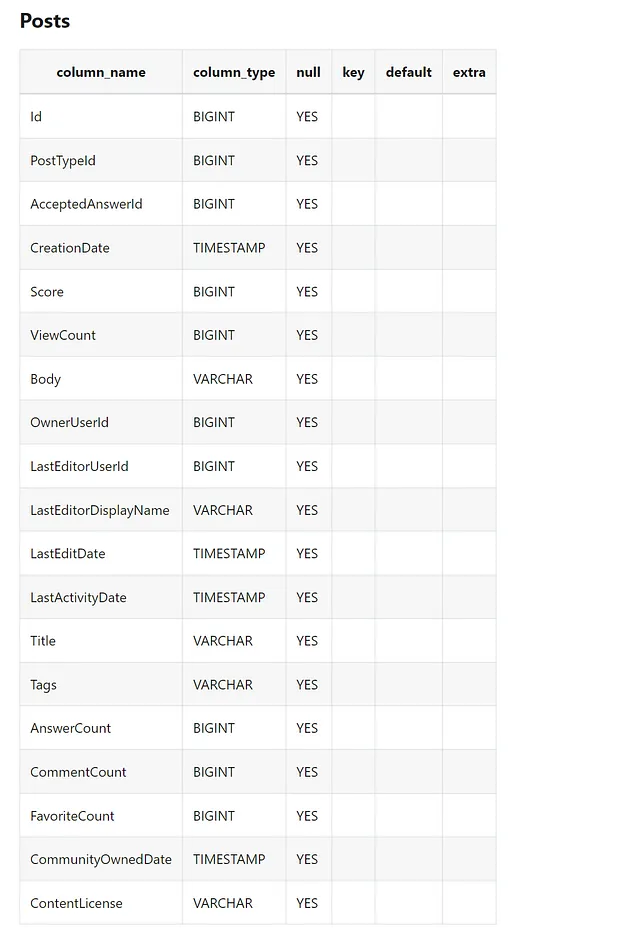
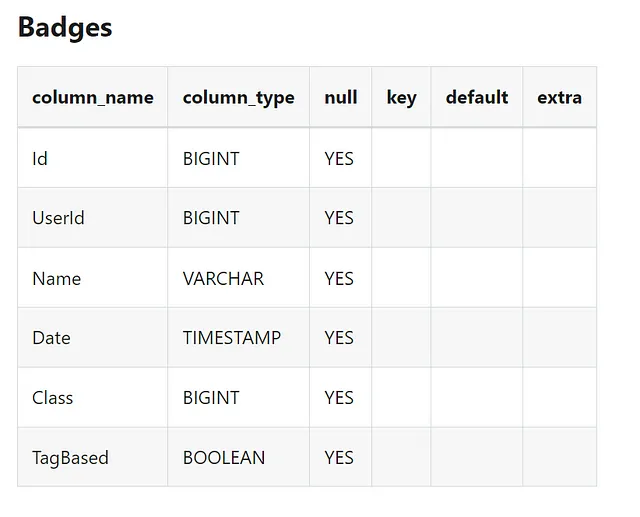
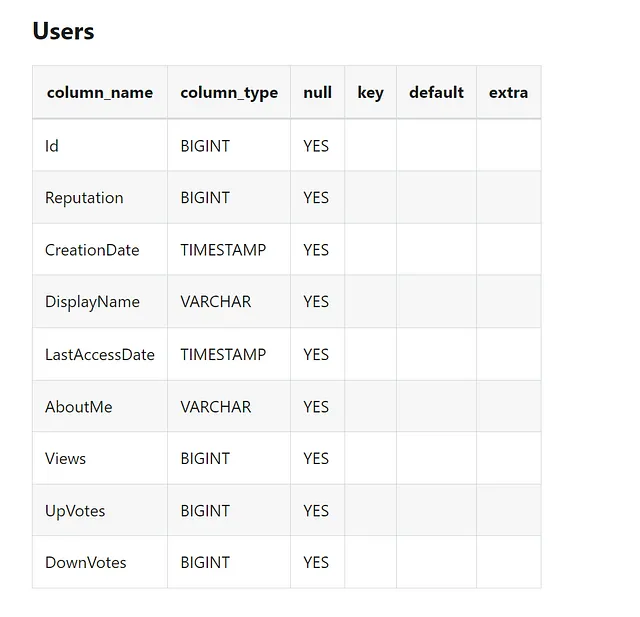
培训计划(信息模式)
# The information schema query may need some tweaking depending on your database. This is a good starting point.
df_information_schema = vn.run_sql("SELECT * FROM INFORMATION_SCHEMA.COLUMNS")
# This will break up the information schema into bite-sized chunks that can be referenced by the LLM
plan = vn.get_training_plan_generic(df_information_schema)
plan
# If you like the plan, then uncomment this and run it to train
vn.train(plan=plan)
Sure, here's the translation in simplified Chinese while keeping the HTML structure: ```html
DDL训练
```# In duckDB the describe statement can fetch the DDL for any table
vn.train(ddl="DESCRIBE SELECT * FROM Stackoverflow.users;")
Sure, here's the translated text in simplified Chinese: ```html
SQL语句培训
```# here is an example of training on SQL statements
vn.train(
question="What are the top 10 users with highest amount of Badges?"
,sql="""SELECT UserId, COUNT(*) AS badge_count
FROM stackoverflow.main.badges
GROUP BY UserId
ORDER BY badge_count DESC
LIMIT 10
""")
# Another example
vn.train(
question="What is the difference in total answers for the user who answered the most answers and the user who answered the least questions?",
,sql="SELECT MAX(answer_count) - MIN(answer_count) AS difference
FROM (
SELECT OwnerUserId, COUNT(*) AS answer_count
FROM stackoverflow.main.posts
WHERE PostTypeId = 2
GROUP BY OwnerUserId
) AS answer_counts;
")
Sure, here's the translation: 培训文档
# You can feed in contextual information using documentation
vn.train(documentation="We call the user with the highest answers in a year the Grand master")
Sure, here's the translation of the English text "You can view your training data using vn.get_training_data()" into simplified Chinese while keeping the HTML structure intact: ```html 您可以使用 vn.get_training_data() 查看您的训练数据。 ```
# vn.ask is runs these following functions in sequence, which can be run individually
# 1. vn.generate_ql
# 2. vn.run_sql
# 3. vn.generate_plotly_code
# 4. vn.get_plotly_figure
# this is how you can ask Vanna question's post training
vn.ask('Find the top 10 users with the highest amount of Badges?')
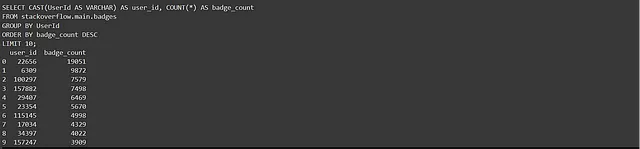
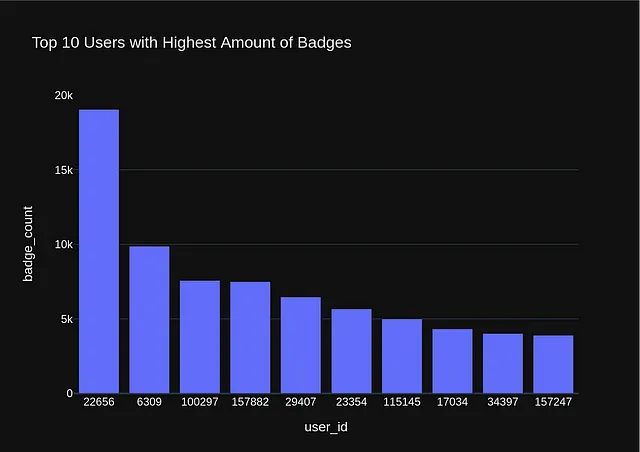
使用 Flask 应用程序
Sure, here's the translation in simplified Chinese while keeping the HTML structure: ```html
Vanna自带一个内置的UI Flask应用程序。可以在Jupyter笔记本或Python脚本中启动。
```from vanna.flask import VannaFlaskApp
app = VannaFlaskApp(vn)
app.run()
Sure, here's the translated text in simplified Chinese: 这是您如何使用 GPT 4o 进行文本到 SQL 的方法。
Benchmark 基准
以下是GPT 40与其同类模型的比较,你可以看到它的准确率为61%,而ChatGPT 4 Turbo为59%,Claude Opus为56%。
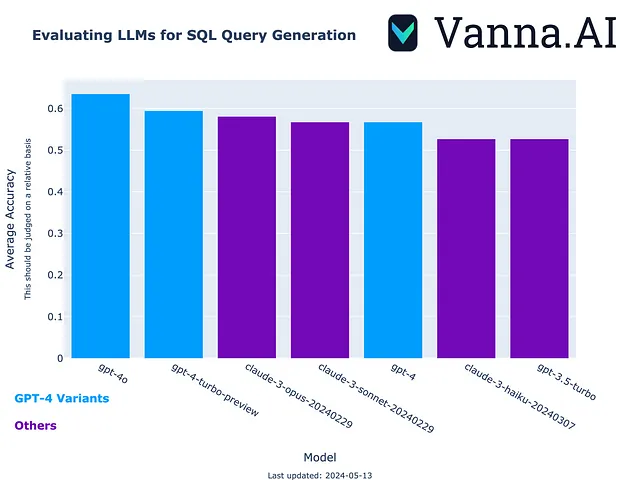
Sure, here is the translated text in simplified Chinese: 感谢您的阅读!